The IRS and Data Science
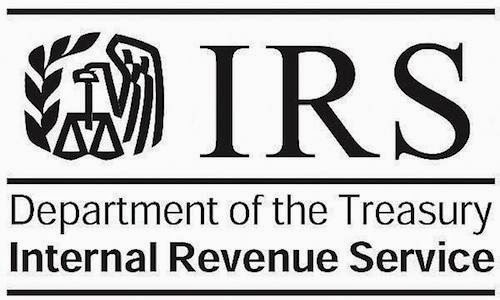
Today, the NYT publishes an article about the IRS seizing bank accounts on suspicion. In short, the IRS intends to battle drug cartels by flagging accounts with a suspicious activity. The quote from the officer gives us an insight about their methodology: - based on my training and experience, the pattern “is consistent with structuring.” In other words, Drug Cartels have a higher propensity to make deposits below $10K (legal ceiling before the bank reports it by law) thus the IRS flagged all of the accounts fitting this criterion. However, the stats reported by Institute for Justice are reported to be mediocre: Only one in five was prosecuted as a criminal structuring case If innocent, the victims are left to prove it with all the red-tape it involves. The problem: This method simply does not work well for the following reasons: 1- A False Positive (Seizing an account that did not belong to Drug Organization) is very costly, from a reputation persp